SAS Healthcare Startup
-
Healthcare is a huge industry, and one in which we’re invested for the short and long term. We want to help make a tangible difference to service through the use and implementation of data analytics in healthcare systems. Recent strikes from the frontline staff highlight the urgency of change needed in the working environment, to implement better processes and curtail the commonplace workarounds conducted and ensure the best service to patients is maintained.
Implementation of software solutions and correct analytics capabilities can overcome many difficulties faced by healthcare professionals, yet it is often overlooked as a non-essential requirement in the business-as-usual state. However, the time afforded to healthcare professionals through the implementation of modern data automated solutions has exponential positive knock-on effects.
-
We have partnered with SAS to enable a time-bound and cost-bound collaborative approach. In 3 months, our POC delivery aims to prove valuable use cases for analytics modelling in NHS trusts. Through a range of services we provide, our aim is to use the most relevant software solution to innovate medical practice within the data analytics space.
Regardless of where you are on your analytics journey, you can achieve breakthrough results.
Clinical Use Cases
-
Natural Language Processing of medical notes
Categorise injuries within accident records to uncover chronological patterns and trends within geographical regions. The integration of natural language processing (NLP) enables more intelligible insights to be uncovered at rapid pace, encouraging proactive over reactive behaviours.
-
Assemble clinical/chronic events quickly
By using fully documented logic and clear logical business rules, clinincal teams can focus on conveying outcomes to analysts and clinicians at rapid pace. Live streaming of data input cannot be achieved whilst remaining reliant on repetative manual processes. Save time and money with intelligent automated interventions.
-
Compare results and costs by visualising patterns in health data
Identify relationships between demographic subsets and clinical events using point-and-click interfaces, to better understand variances in health outcomes, be that within a provider network or clinical study.
-
Standardise clinical data efficiently
Create libraries of validated data mappings that convert source entities into standard input processes that fulfil clinical and regulatory requirements. The mappings may be used for recurring transformations, improving efficiency and accuracy accross the business.
Are you ready to take your analytic journey to the next level?
Using prebuilt capabilities, you may easily integrate unstructured and streaming data to your structured data. Text analytics, computer vision, and natural language processing may all be included in your predictive models. You may lay the groundwork for repeatable, industry-specific applications and creative AI solutions that can assist you in effectively navigating market change.
Here are some of the projects we have worked on.
-
Bedspace management.
Using predictive analytics and historic data to better understand the likely medium-term requirements for bed space numbers according to inpatient observations and biometrics. See our bed space management proof of concept hackathon here.
-
Chatbots
We have built a Cancer Information helpdesk in the form of a Chatbot as part of our final submission. For those wanting access to information from reliable sources, our Chatbot uses AI to provide an efficient yet familiar service to family and friends of cancer patients.
-
Natural Language Processing
COVID-19 patient data was used to create a model that predicts whether a patient is likely to be a short or long-term patient in the hospital, based on readings of vital signs and other variables. This results in accurate clinical decision-making and further resource allocation.
-
Adjust for risk and severity in population health or claims data efficiently.
Allow for risk adjustment via repeatable and transparent methods. Models included inside the platform assist analytic leaders in understanding population-level cost drivers, comorbidities, and projected outcomes for individual episodes of treatment. Financial analysis helps risk managers as well as physicians who aim to improve treatment approaches.
-
Analyse and visualise cohort data.
We can create an interactive, drag-and-drop interface for searching and constructing temporal cohorts. To determine research feasibility, you may quickly investigate cohort features and the influence of inclusion/exclusion criteria on patient populations. You may retain cohort definitions for later use, alter them, and apply them to other real-world data assets for population comparisons, saving time and resources. Validate results and do additional research on your preferred technologies (e.g. R, Python and third-party visualisation tools) utilising in-memory analysis and visualisation.
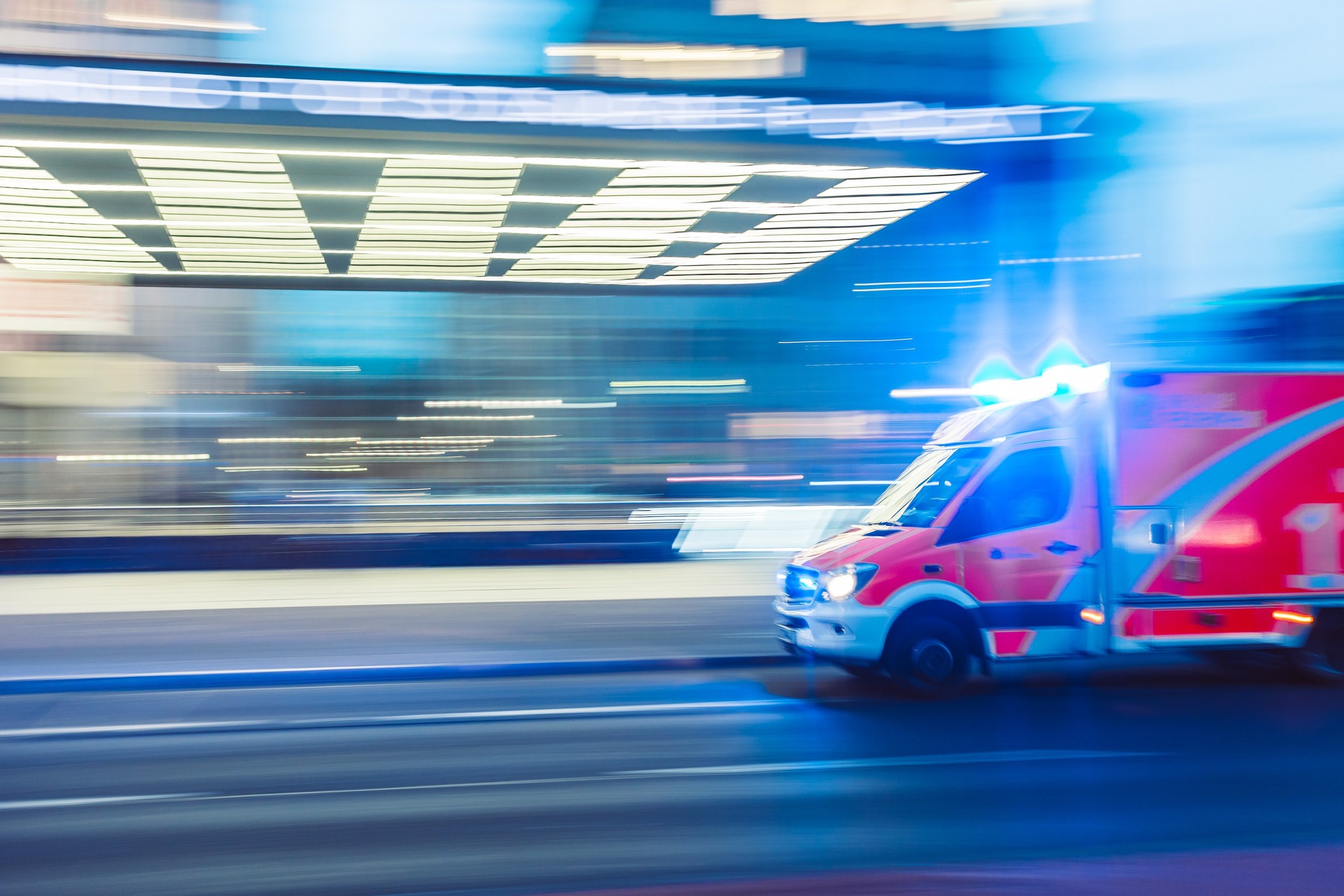